
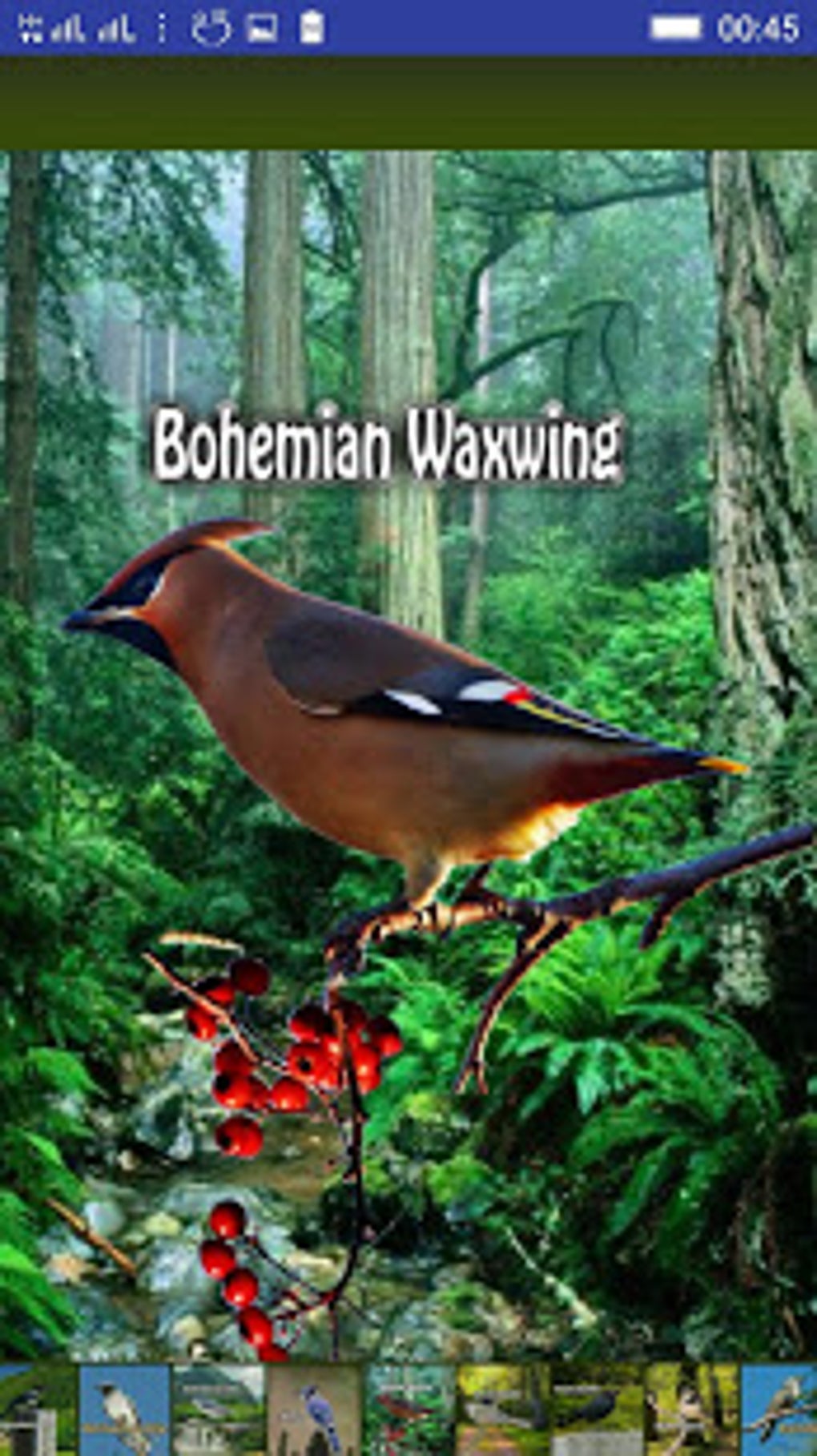
Remote monitoring night-flight calls, Poland ("PolandNFC") - 4,000 recordings from Hanna Pamuɫa's PhD project of monitoring autumn nocturnal bird migration. The audio covers a range of birds and includes weather, large mammal and insect noise sampled across various CEZ environments, including abandoned village, grassland and forest areas. This data was collected as part of the TREE (Transfer-Exposure-Effects) research project into the long-term effects of the Chernobyl accident on local ecology. Remote monitoring dataset, Chernobyl ("Chernobyl") - 6,620 audio clips collected from unattended remote monitoring equipment in the Chernobyl Exclusion Zone (CEZ). (Thanks to Internet Archive, Zenodo and Figshare for dataset hosting) Evaluation datasetsĬrowdsourced dataset, UK ("warblrb10k") - a held-out set of 2,000 recordings from the same conditions as the Warblr development dataset. Remote monitoring flight calls, USA ("BirdVox-DCASE-20k") - 20,000 audio clips collected from remote monitoring units placed near Ithaca, NY, USA during the autumn of 2015, by the BirdVox project. The audio covers a wide distribution of UK locations and environments, and includes weather noise, traffic noise, human speech and even human bird imitations.
#All about birds sounds manual#
Note that there will in general be some low level of error/disagreement in "ground truth" manual labelling.

We provide three development datasets, and three evaluation datasets, each from a separate bird sound monitoring project.Īll of the datasets contain 10-second-long WAV files (44.1 kHz mono PCM), and are manually labelled with a 0 or 1 to indicate the absence/presence of any birds within that 10-second audio clip. The evaluation will also consider each dataset separately and combine the outcomes, rather than treating them as a single pooled dataset.
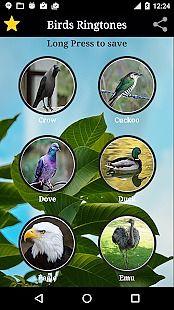
This means that adapting to the overall characteristics of each dataset separately is possible. Note that for every audio clip, you will be told which dataset it belongs to. To solve this task well, you will need an approach which either inherently generalises across conditions (including conditions not seen in the training data), or which can self-adapt to new datasets ("domain adaptation"). The datasets will have different balances of positive/negative cases, different bird species, different background sounds, different recording equipment. To explore this we provide 3 separate development datasets, and 3 evaluation datasets, each recorded under differing conditions. The output can be just "0" or "1", but we encourage weighted/probability outputs in the continuous range for the purposes of evaluation.įor the main assessment we will use the well-known "Area Under the ROC Curve" ( AUC) measure of classification performance.Īn important goal of this task is generalisation to new conditions. The task is to design a system that, given a short audio recording, returns a binary decision for the presence/absence of bird sound (bird sound of any kind). This task is an expanded version of the Bird Audio Detection challenge which ran in 2016/2017. continuous 24h monitoring) by filtering data down to regions of interest. classification, counting), and makes it possible to conduct work with large datasets (e.g. Bird sound detection is a very common required first step before further analysis (e.g. Detecting bird sounds in audio is an important task for automatic wildlife monitoring, as well as in citizen science and audio library management.
